Home
Welcome to the Safe-Drive IV Workshop 2024!
Ensuring the safety of autonomous vehicles is a complex and intricate task that necessitates a comprehensive approach across various domains. Given the prohibitive costs of real-world testing, simulating edge scenarios emerges as a viable solution to ascertain the safety of AVs. However, traditional simulation methodologies often impose a trade-off between realism and controllability, creating substantial discrepancies in accurately mirroring the complex dynamics of real-world driving scenarios. Additionally, these data-driven simulations tend to neglect the complex network of multi-agent interactions that AVs must navigate, a critical factor influencing both safety and operational efficiency. This requires a holistic approach to simulation, one that incorporates the subtle interactions between various road users and environmental conditions, ensuring the evolution of robust and reliable AV systems.
Contact: iv2024safeav@gmail.com
Call For Papers
This workshop aims to foster advancements in AV safety and adaptability by delving into innovative, data-driven simulation methodologies and exploring the complexities of multi-agent interactions. The topics of interest within the scope of this workshop include, but are not limited to :
• Generating data-driven traffic simulations for autonomous vehicle (AV) safety
• Pedestrian prediction and its effect on AV safety
• Transparency and explainability of vehicle-pedestrian interactions in multi-agent environments
• Transferable and continual driver behavior modeling
• Adversarial testing and validation frameworks, adversarial attacks, and falsification of autonomous vehicle (AV) sub-modules (e.g., perception, decision-making, communication, and control)
• Applications of AVs interacting with human agents
• Naturalistic driving environment modeling with statistical realism
Keywords
AV safety;
Keynote Speakers
-
Yulong Cao
Research Scientist
Nvidia, USA -
Heejin Ahn
Assistant Professor
Korea Advanced Institute of Science and Technology, South korea -
Rowan McAllister
Staff Research Scientist
Waymo, USA
-
Elin Anna Topp
Associate Professor
Lund University, Sweden -
Maximilian Naumann
Research Scientist
Bosch, Germany -
Hyo-Sang Shin
Professor
Korea Advanced Institute of Science and Technology, South korea
Schedule
June 2nd, 2024 in in Halla Room B. All times are in Korean Standard Time (KST). Current time is . The keynote speaker has 15 minutes for their talk, followed by 5 minutes for Q&A. For paper presentations, there will be 8 minutes allocated for oral presentation and 2 minutes for questions and answers.
Submissions
Workshop Code for submission: SAFE-DRIVE
Workshop Paper Submission deadline: 1st February 2024 at 23:59 Anywhere on Earth
Workshop Paper Notification of Acceptance: March 30, 2024
Workshop Final Paper Submission Deadline: April 22, 2024
Paper submission here.
FAQs
Q: Will there be archival proceedings?
A: Yes, All submitted manuscripts will be peer-reviewed and will be published in IEEE proceedings.
Q: Should submitted papers be anonymized or without authors names?
A: No.
Q: My paper contains ABC, but not XYZ, is this good enough for a submission?
A: Submissions will be peer-reviewed based on the information provided here.
Q: My question is not listed here. How can I contact you?
A: Please reach out to us at the email address iv2024safeav@gmail.com and we'll be happy to answer any questions you have!
Q: Will the deadline for paper submission be extented?
A: We are afraid that all deadline are firm and will not be extended.
Q: What is the unique code for this workshop for submission?
A: The unique code for submitting the paper for this workshop is "SAFE-DRIVE". More Information here.
Keynote Talks
Next-Generation Testing for Autonomous Vehicles: A Focus on Behavior and Simulation
Yulong Cao
Abstract
Ensuring the security and safety of autonomous vehicle (AV) driving systems is a critical challenge due to the inherent safety risks. Efficiently testing AVs in real-world scenarios remains unresolved due to the complex and varied nature of driving behaviors and semantics. In this talk, I will present recent research efforts aimed at rethinking the testing of AV systems. The discussion will focus on two key areas: [1] modeling diverse behaviors using advanced traffic models, and [2] leveraging foundation models to enhance simulation capabilities and accelerate the testing process.
Behavior Models for Interactive AV Forecasting and Planning
Rowan McAllister
Abstract
TBD
Supporting Users Situation Awareness for Remote Control of (almost) Autonomous Vehicles
Elin Anna Topp
Abstract
In this talk I will give an overview over some recent and ongoing projects, that all in some way aim at providing situational awareness or comprehensible tools for remote control or surveillance of different types of (autonomous) agents: Unmanned surface vessels, mobile robotic platforms, cars, and drones/fixed wing unmanned aircraft.
In all cases the idea is that we try to provide these systems with the means to get input (e.g. instructions or corrections) from users that can actually support them in their task performance. To get this meaningful information, users need to be supported themselves, based on the assumption that a non-expert user (non-expert regarding a vehicle's or other type of system's internal functionality) might have difficulties to provide vehicle adapted information easily. Hence, we want to equip our systems with tools to provide situational awareness and with intuitively useable interfaces, that support the users in instructing and giving information to the system as needed and as deemed suitable.
Generative Behavior Modeling for AD Simulation
Maximilian Naumann
Abstract
Conducting real-world testing of autonomous vehicles on the road can be costly and risky, and does not scale, which is why simulation is a vital tool for speeding up the advancement of safe autonomous driving technology. However, in order to reduce the disparity between simulation and reality, it is essential to have effective agent behavior models. This talk will give an introduction to closed-loop training of sequential decision networks for generative behavior modeling, and teaser recent approaches to leverage vision and language foundation models for this task.
Intent-Informed State Estimation for Tracking.
Hyo-Sang Shin
Abstract
TBD
Safe Autonomous Driving under Perception Uncertainties
Heejin Ahn
Abstract
Failures of autonomous driving systems are still common, often leading to catastrophic consequences. A significant number of these failures stem from the inherent fragility of data-driven perception modules. In this talk, I will focus on control techniques that guarantee the safety of autonomous vehicles in the presence of perception uncertainties. Specifically, I will present several designs of stochastic control, which use probabilistic methods to account for uncertainties in predicting other vehicles behaviors and identifying traffic signs. Finally, I will discuss future research directions for advancing the safety and performance of autonomous vehicles.
Accepted Papers
Full Papers
Pedestrian Groups Matter: Unraveling their Impact on Pedestrian Crossings when Interacting with an Automated Vehicle
Maximilian Hübner, Martina Baude, and Klaus Bengler
Abstract
Since future mobility will change into mixed traffic,the communication approach of automated vehicles should consider multiple human receivers. This virtual reality study investigates the external communication of an automated vehicle
in interactions with multiple pedestrians. Involving 42
participants, the study explores the impact of a simulated
pedestrian group at the roadside, a seldom-explored aspect in
current research on human-vehicle interactions. Results reveal
a significant influence of the additional pedestrian group on
participants' behaviors and perceptions, emphasizing the need
for nuanced communication strategies in automated vehicle
design. This paper contributes to understanding social factors in
automated vehicle interactions, providing valuable insights for
future research and the development of human-centric
automated vehicle communication systems.
Pedestrian Safety by Intent Prediction: A Lightweight LSTM-Attention Architecture and Experimental Evaluations with Real-World Datasets
Afnan Alofi, Ross Greer, Akshay Gopalkrishnan and Mohan Trivedi
Abstract
Autonomous vehicles face significant challenges in
understanding pedestrian behavior, particularly in urban environments. In such settings, the system must recognize pedestrian
intentions and anticipate their actions to achieve safe and
intelligent driving. This paper focuses on predicting pedestrian
crossings, enabling oncoming vehicles to react to pedestrians in a
traffic scene in a timely manner. We investigate the effectiveness
of various input features for pedestrian crossing prediction,
including human poses, bounding boxes, and ego vehicle speed
features. We propose a novel lightweight architecture based on
LSTM and attention to accurately identify crossing pedestrians.
Our methods are evaluated on two widely used public datasets for
pedestrian behavior, PIE and JAAD datasets, and our algorithm
achieves a state-of-the-art performance in both datasets by
reaching a prediction accuracy of 91% and an F1-score of
84% on the PIE dataset and an accuracy of 67% and an F1-score of 77% on the JAAD Behavior (BEH) split.
In Search of Social Presence: Evoking an Impression of Real Pedestrian Behavior Using Motion Capture
Chantal Himmels, Jakob Peintner, Carina Manger, Teresa Rock, Oliver Jung,and Andreas Riener
Abstract
Virtual Reality (VR) is commonly utilized to examine driver interactions with vulnerable road users
(VRUs) in an effective and secure manner. Recent studies,
however, have highlighted issues in VR simulations, particularly concerning the authenticity of state-of-the-art pedes-
trian agent behaviors. These inaccuracies can compromise
the perceived realism of the situation, potentially leading to
unrepresentative driver reactions. This paper aims to showcase enhancements in pedestrian agent models and evaluate
their subsequent advantages. To this end, real pedestrian
movements, captured via motion-capture technology, were
compared with outputs from a contemporary pedestrian
agent model within a VR driving simulator experiment. The
findings underpin the advantages of using motion-captured
pedestrians to enhance social presence. Additionally, participant feedback emphasized that certain elements, such as
head movements, explicit gestures, and subtle cues like hesitation before entering the road, were crucial in distinguishing
realistic from unrealistic agents. These insights contribute
significantly to refining the focus for systematic advancements
in (pedestrian) agent models in VR environments. Such improvements are pivotal in augmenting the users sense of
presence and the behavioral accuracy of the simulations.
Connected and Automated Transportation System in Multi-Agent Environment
Angah, Ohay, Zhang, Yiran and Ban, Xuegang (Jeff)
Abstract
Traffic simulation is important for transportation
researchers, analysts, and policymakers. It can be used to test
vehicle/traffic control algorithms, gain insights into traffic
dynamics, and develop traffic management strategies that can
improve the efficiency and safety of transportation systems.
Unfortunately, many existing simulation platforms have
limitations to cater to diverse simulation scales. This study
presents a comprehensive multiscale vehicle-traffic-demand
(VTD) simulation platform tailored for connected and
automated transportation systems. This platform integrates
Unity 3D, Simulation of Urban Mobility (SUMO), and
Multiagent Transport Simulation (MATSim) to facilitate an in-depth analysis of both micro and macro-level traffic behaviors.
A critical aspect of our work involves the meticulous setup and
calibration of traffic networks in Greater and Downtown Seattle,
ensuring effective integration and communication between the
various simulation tools. This advanced platform not only serves
as a robust tool for testing and refining vehicle/traffic control
algorithms but also opens new avenues for research into traffic
dynamics learning and the development of sophisticated traffic
control solutions.
Organizers
-
Shoaib Azam
is a Postdoctoral Scholar at Aalto University & Finnish center for Artifical Intelligence, Finland.
-
Tsvetomila Mihaylova
is a Postdoctoral Scholar at Aalto University, Finland.
-
Stefan Reitmann
is a research fellow at Lund University, Sweden.
-
Farzeen Munir
is a Postdoctoral Scholar at Aalto University & Finnish center for Artifical Intelligence, Finland.
-
Syed Arsal
is a Postdoctoral Scholar at RTIS lab University of Nevada Las Vegas (UNLV), USA.
-
Nico Uhlemann
is a PhD student at the Technical University of Munich, Germany.
Advisory Committee
-
Ville Kyrki
Associate Professor
Aalto University, Finland -
Tomasz Kucner
Assistant Professor
Aalto University, Finland
-
Moongu Jeon
Professor
Gwangju Institute of Science and Technology, South Korea -
Laura Ruotsalainen
Professor
University of Helsinki, Finland
Partners
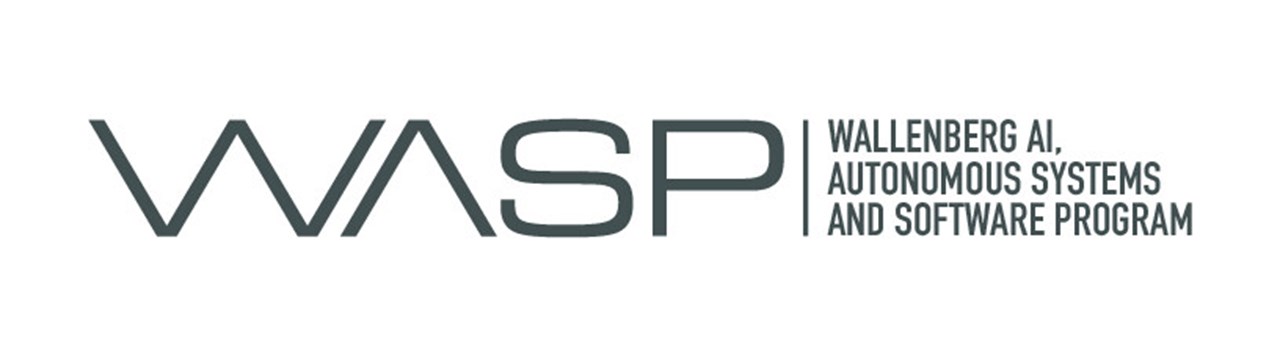
Location
Theater B, 2nd floor Landing Convention Centerr, 38 Sinhwayeoksa-ro 304 beon-gil, Andeok-myeon Seogwipo-si, Jeju, South Korea